Table of Contents
ToggleData Engineering
Data engineering aims at making the data received and generated across various information systems of the enterprise useful and accessible. In the context of Sourcing and Procurement Analytics, its primary objective is to improve the reliability, accessibility, and coherence of the data so that slicing and dicing across disparate dimensions become possible resulting in useful and not-so-obvious insights from the data.
Data engineering is the process of collating, transforming and relating data of different business process systems into a shared repository with secured access to appropriate stakeholders.
Typical Sourcing Systems landscape
Sourcing has evolved into a critical business process over the last few decades. What started as a means to procure materials and services in the most cost-effective method has transformed into a C-suite skill that is pivotal for sustainable business success.
Along with the evolution of Sourcing, information systems designed to execute different business processes continued to evolve too. Such evolution was primarily horizontal with the induction of several IT applications specializing in specific areas of sourcing into the Enterprise IT landscape.
Today, the IT ecosystem of the Sourcing process in large enterprises typically looks like this:
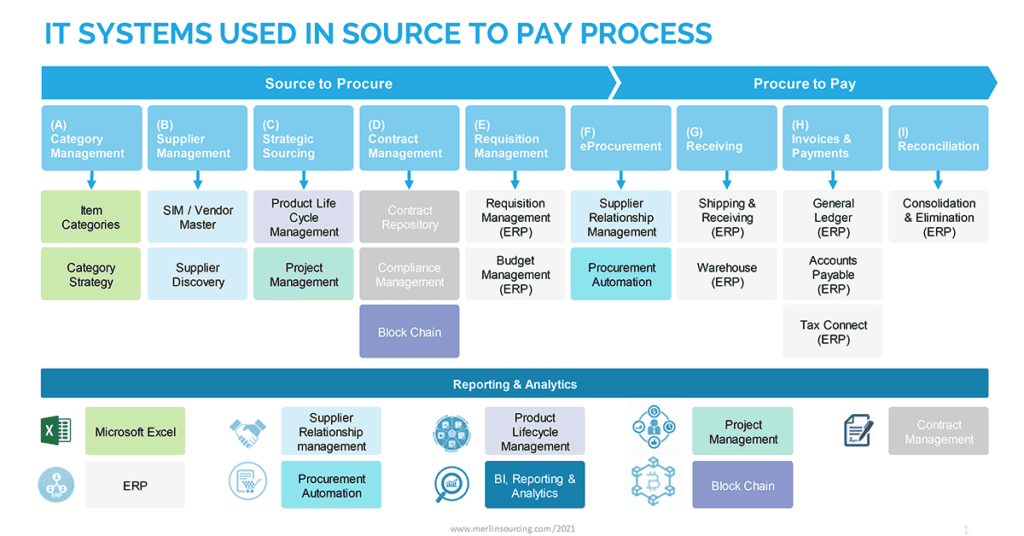
It becomes obvious from the picture that the ecosystem consists of disparate systems coming from different technologies working together to meet the business process goals.
The Implication of a Disparate ecosystem
An ecosystem with such several IT applications from technologies from different periods working together brings its own challenges including the following:
- Lack of end-to-end automation
- Lack of cohesive User experience
- Missing business process steps
- Lack of agility to respond to changing business needs

Another important implication of this ecosystem is the lack of complete, correct, and cohesive data to make timely and correct business decisions.
Implication on Data
Data generated from such systems usually have the following challenges:
Time periods do not match
- One of the systems can generate weekly data while another can only bring out monthly data.
Transaction integrity does match
- While a purchase ordering system may have all orders including incomplete ones, a customer-facing system will need only accepted orders.
Perspectives of dimensions do not match
- Supplier’s geographical regions may differ in how orders are grouped by regions.
Unique identity of business entities does not match
- For example, a supplier may be identified by a unique number in the Supplier Relationship Management system. However, the supplier maybe identified using a different identifier in your PO management system. Now, since the data of business entities (in the above example, supplier) exists in multiple systems, it can be challenging to find all records that pertain to the entity. There may not be a unique identifier for every business entity to indicate which entity from one system correspond to those in other systems.
Missing data elements
- A data key used to combine more than one entity may be missing
These challenges create data silos making them complex to bring such unreliable data structures together to make a meaningful impact.
Significance of Data Engineering
These challenges make Data Engineering a significant component of making data relevant and complete for sourcing and procurement analytics. Data Engineering is the process of aligning all possible elements of data entities across each other so that meaningful insights can be derived from them.
Data Engineering also involves the step of finding missing elements in data entities and replacing them with meaningful default values.
Data Engineering Challenges
Along with the needs and benefits of Data Engineering, there are challenges as well:
- Data Engineering takes an inter-disciplinary effort of combining both business and technology teams to understand challenges and bridge data gaps – It is hard to get to this
- It is hard to imagine every possible data gap upfront and design – Design needs to be responsive and agile and quickly address challenges as they pop-up
- Data Engineering is a time-consuming process both in design and execution – Adds a significant lag between data origin and its availability for analytics
- Data Engineering is based on several assumptions – These assumptions reduce the quality of data and hence the quality of analytics too
- Connected systems of the ecosystem go through changes – Data Engineering assumptions continuously get impacted and hence need continuous changes as well
Need for a unified Sourcing Solution
Though Data Engineering is one of the steps required to make sure that data from disparate systems talk to each other, no amount of Data Engineering effort will be an effective replacement for a cohesive system that collates all data internally.
RheinBrücke’s MeRLIN is a Sourcing Solution for Direct & Indirect Procurement that offers a seamlessly integrated sourcing process automation with supplier relationship management and planning functions augmented by advanced analytics. It enables digital transformation of your procurement function, progressing from supporting cost control to driving strategic sourcing initiatives. It provides a platform to clearly understand spend, identify sourcing needs, successfully define and execute sourcing strategies using data driven insights, manage suppliers, RFx and eAuctions, and simplify decision-making using clear actionable analytics.
Contact us to know more.
3 comments
Nice post. Waiting for your next article.
Thanks for your comment. We are glad that you find our posts interesting!
Thank you for the comment. We are glad that you found this post interesting!